topical media & game development
PDF
EXPERT ADVICE AND REGRET FOR SERIAL RECOMMENDERS
Anton Eliëns, Yiwen Wang
abstract
In this paper we propose a tentative framework (R3) for adapting
a sequence of predictions (guided tour) generated by what we call a serial recommender.
The R3 framework (rate, recommend, regret) is applied to the construction
of personalized guided tours, based on expert advice, in the domain of cultural heritage,
in particular digital dossiers about contemporary art.
Guided tours are in first instance obtained by tracking expert users.
Our proposal is based on a variant of decision theory,
that uses a regret function to measure the difference between
a proposed decision and a finite collection of expert decisions.
In our framework, personalization may then be seen as a minimization
problem over a weighting scheme, expressing the relative importance
of experts of which tours are available.
Our aim in this paper is to arrive at a formalization of the recommendation
of sequences (guided tours) that allows for adaptation to individual user preferences
by a revision of the weight attached to a particular advice based on user feedback.
keywords:
decision theory, personalization, guided tours, digital dossier, cultural heritage
INTRODUCTION
Leaving all responsibility for interaction to the user
is usually not a good choice, in particular when an information system contains
complex, highly interrelated information.
Despite the wealth of recommendation systems, it still seems
to be an open problem how to generate a related collection
of recommendations, that is an organized sequence of
recommended items that me be used as a guided tour,
for example an overview of artworks and related information
from a museum collection.
In [Eliens et al. (2006b)], [Wang et al. (2006)], [van Riel et al. (2006)] we describe the 3D digital dossier format,
in which we presented the information of respectively the Dutch-Serbian artist
Marina Abramovic and the Australian
artist Jeffrey Shaw,
contemporary artists with a variety of work, ranging from video to art installations.
The digital dossier supports navigation using a concept graph and allows
for presenting media-rich material, including 3D models of artwork installations.
The digital dossiers have been implemented using X3D/VRML
to allow for deployment on the web.
Recently we have explored guided tours in digital dossiers, [van Riel et al. (2006)],
which actually automate user interaction, by mimicking user actions through events generated
by a script.
Although this provides an easy way to create guided tours, this does not solve the problem
of what to select as elements in the guided tour, or how to personalize
these tours in an intelligent manner.
In this paper, we discuss techniques from decision theory
as a means to aid the construction of guided tours by consulting an advice
function based on tracking the navigation behavior of expert users.
We will also indicate how a similar advice function can be used for
personalizing tours in cooperation with a recommender system for artworks,
by altering the weight given to particular properties.
More in general, our aim is to arrive at a formalization of the mechanics underlying the recommendation
of sequences (guided tours) that allows for adaptation to individual user preferences
by a revision of the weight attached to a particular advice based on user feedback.
Moreover we will give an indication how to generalize our approach to include the
refinement of content-based ratings from which sequences are generated, by adapting
weight attached to specific attributes of items featured in the guided tour.
We opt for the phrase serial recommender, to stress on the one hand that
the recommendation concerns sequences and not individual items, and on the other hand
what one may call the compulsive nature of the recommendations, due to the fact
that they are originally generated by experts.
Mind that our approach has been primarily motivated by the need to support guided tours
in digital dossiers. As we discuss in more detail in the paper, digital dossiers, and
in particular the concept graph as a navigation paradigm, adhere to specific constraints
that do not apply in general.
As a consequence, it might be hard to generalize the approach to other domains where
guided tours are useful. However, by including ratings based on content and an appropriate
distance function between recommended items, it seems that
the R3 framework introduced here is applicable to a wider class of (serial) recommenders.
structure
The structure of this paper is as follows.
First we will give a brief overview of recommdender systems, after
which we will give a short introduction to decision theory.
Then we will describe the abramovic dossier,
and discuss how techniques from decision theory can be
applied to the construction of guided tours in digital dossiers,
followed by a discussion
of how to realize expert advice functions in digital dossiers.
We will then
illustrate how to apply decision theory for the personalization of
tours in a more conventional cultural heritage application,
sketch a formal model for (serial) recommender systems,
introduce a distance function for item recommendations, and indicate how
to deal with user feedback discrepancy.
Finally, we will
give our conclusions and indicate directions for future research.
RECOMMENDER SYSTEMS -- BRIEF OVERVIEW
There is a great wealth of recommender systems,
and a daunting number of techniques for producing recommendations, based on content,
user behavior or social groups. See the AAAI 2004 Tutorial
on recommender systems and techniques for an (extensive) overview.
In [Van Setten (2005)] a distinction is made between the following types of prediction techniques:
prediction techniques
- social-based -- dependent on (group) rating of item(s)
- information-based -- dependent on features of item(s)
- hybrid methods -- combining predictors
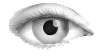
Social-based prediction techniques include collaborative filtering (CF),
item-item filtering, popularity measures, etcetera.
Information-based prediction techniques include information filtering,
case-based reasoning and attribute or feature comparison.
Finally, as hybridization techniques, [Van Setten (2005)] distinguishes
between weighted combination, switching, mixed application and
meta-approaches such as feature combination and cascaded application.
The approach we present in this paper,
the R3 framework, has aspects of social-based as well as information-based methods
and may be characterized as hybrid since it uses a weighting scheme to select between
experts for advice.
For clarity, it is worthwhile to delineate briefly
what we understand by the phrases rate, recommend, regret,
and how the R3 framework fits within the wider scope of recommendation techniques:
definition(s)
- rating -- a value representing a user's interest
- recommendation -- item(s) that might be of interest to the user
- regret -- a function to measure the accuracy of recommendations
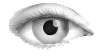
In our approach, we (initially) proceed from the assumption that a
rating is already present,
and more in particular a rating that implies a sequential order on the presentation
of a (limited) number of items. Later, however,
we will explore how to relax this assumption and apply
the R3 framework to sequences that are generated on the basis of
content-based user preferences, to allow for an incremental adaptation of recommendations.
MATHEMATICAL PRELIMINARIES -- DECISION THEORY
Before discussing how to realize guided tours in digital dossiers using user tracking
and expert advice,
we will give a very brief introduction
to decision theory, more in particular a variant of
decision theory introduced in [Cesa-Bianchi and Lugosi (2006)], that
provides a mathematical foundation for our approach.
In classical prediction theory a prediction is a sequence
of elements that results from a stationary stochastic
process.
The risk of the prediction is taken to be the expected value
of the accumulated loss function, measuring the
discrepancy between predicted values and actual
outcomes.
[Cesa-Bianchi and Lugosi (2006)] introduce a variant of prediction theory in which
no assumption is made with respect to the nature of the
source of predictions.
Instead, the forecaster is considered to
be an entity that gives a prediction for an element
based on advice of one or more experts.
These experts might be actual sequences stored in a database.
The deviation of the forecaster with the actual
outcome is measured using a regret function,
and the prediction task may hence be formulated as
minimimizing the regret function by choosing the
best expert for advice for each element of a prediction sequence.
For example, for the prediction of a bitstring of length n,
the forecaster is a vector of n expert indices,
that give advice for the bitvalue, 0 or 1, in that position.
In the general case, in which we have no information
on the error rate of the experts' advice,
we may use a weighting factor $0 <= %b_{i} <= 1